Budget AI Breakthrough: Researchers Unveil Powerful Challenger to OpenAI's GPT Model for Just $50
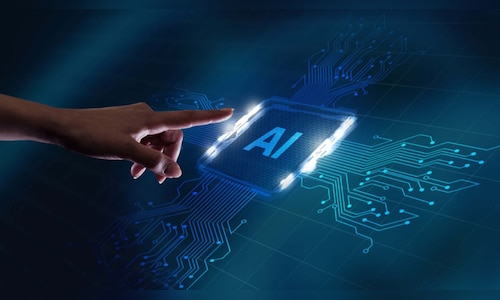
In a remarkable display of computational efficiency, researchers achieved an impressive milestone by training the s1 model in under 30 minutes, leveraging the power of 16 cutting-edge NVIDIA H100 GPUs. This breakthrough highlights the incredible advancements in machine learning technology and computational speed, demonstrating how state-of-the-art hardware can dramatically reduce model training times. The rapid training process not only showcases the model's potential but also underscores the significant progress in artificial intelligence infrastructure.